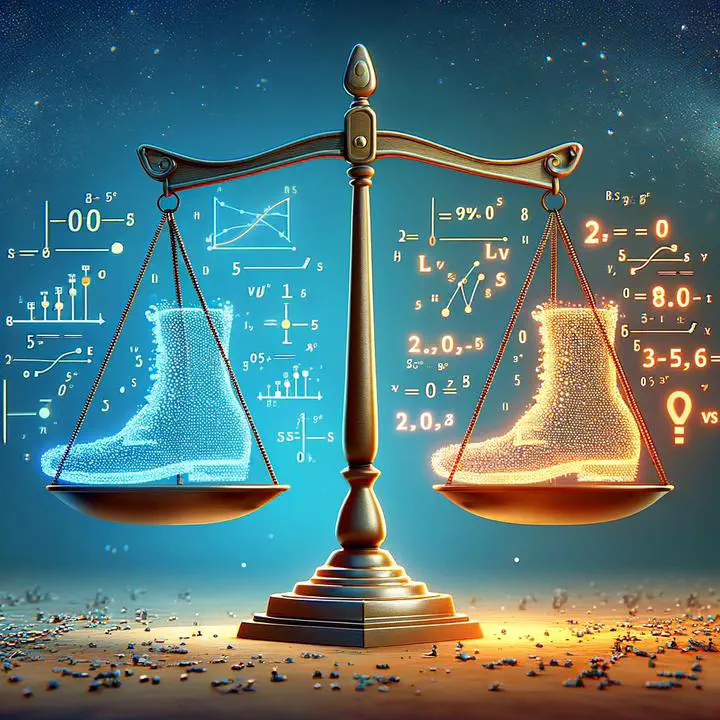
In this project, I generated 15 scenarios of epidemiological confounding data with weak, medium, and strong confounding relationships between covariates and continuous & binary outcomes. I randomly generated 1000 datasets for each scenario to calculate the true effect and variance. I conducted the propensity score matching method via both complex and simple bootstrap to calculate and compare the variability of the average treatment effects with true variance.